Generative AI: A Blessing or a Curse for Cybersecurity?
Delve into the potent intersection of Generative AI and cybersecurity. This article illuminates AI's role in cyber threats and its promise in fortifying digital defenses. Discover the AI-powered future of cybersecurity.
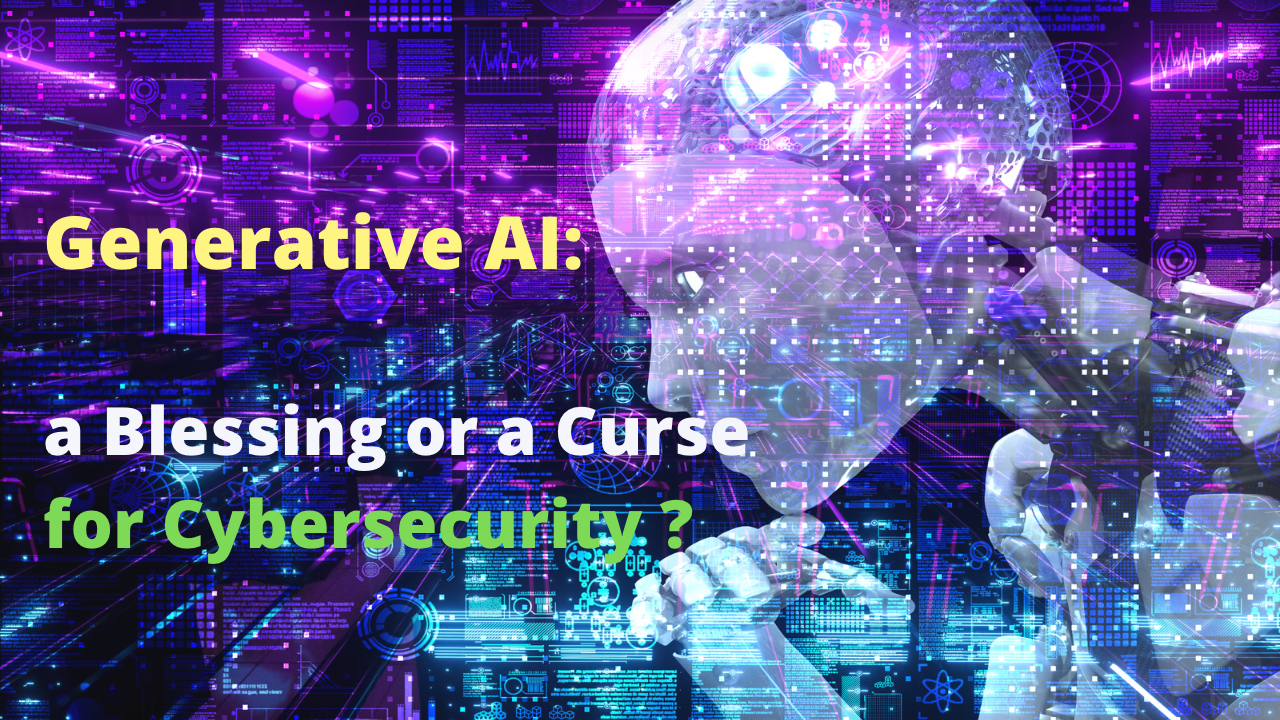
Introduction
Generative AI has emerged as a groundbreaking force within the dynamic landscape of artificial intelligence (AI). With its prowess in crafting remarkably realistic text, audio, video, and even functionally coherent programming code, generative AI has propelled us into a new era of innovation. Large Language Models (LLMs), like ChatGPT, form the vanguard of this AI revolution, their sophisticated algorithms pushing the boundaries of what AI can accomplish. However, with each stride forward, we're compelled to grapple with the implications for cybersecurity—an ever-critical domain dedicated to safeguarding our information systems and networks from unauthorized breaches, thereby reinforcing data privacy and service reliability in our digitized world.
This article navigates the intersection of generative AI and cybersecurity, posing a consequential question: Is generative AI a beacon of progress for our digital future, or could it potentially jeopardize our cybersecurity frameworks?
Security Risks Inherent in Generative AI
Despite the undeniable power and promise of generative AI, it brings its own set of cybersecurity risks to the table.
Data Leakage: A Mounting Concern?
A recent Salesforce survey revealed a sobering fact: 71% of the senior IT leader repsondants think that Generative AI could introduce novel security threats to their data systems. Among these looming risks, data leakage is particularly spotlighted. Data leakage attacks exploit the capacity of generative AI models to yield realistic and coherent text, drawing upon their extensive training data. These attacks aim to coax the model into divulging sensitive or private information, potentially compromising the security and privacy of both users and companies involved with generative AI services.
There are two primary ways in which data leakage can occur:
- Training Data Exposure: generative AI models feed on vast volumes of data for their training. Should this data include sensitive information, there's an inherent risk of the model unintentionally revealing this in its responses. For instance, if confidential business documents or private conversations formed part of the training data, the AI could inadvertently generate outputs that expose this information.
- Input Data Exposure: generative AI models shape their responses based on the input they're fed. In a scenario where a user inputs sensitive data, there's a hypothetical risk of the AI incorporating this data into its output, thereby exposing it to any viewer of the AI's responses.
The risk of input data exposure has piqued especially significant concern among businesses wary of potential internal data leaks through generative AI usage. For example, an April 2023 analysis by Cyberhaven Labs demonstrated that despite apprehensions, the use of ChatGPT in workplaces has been expanding rapidly.
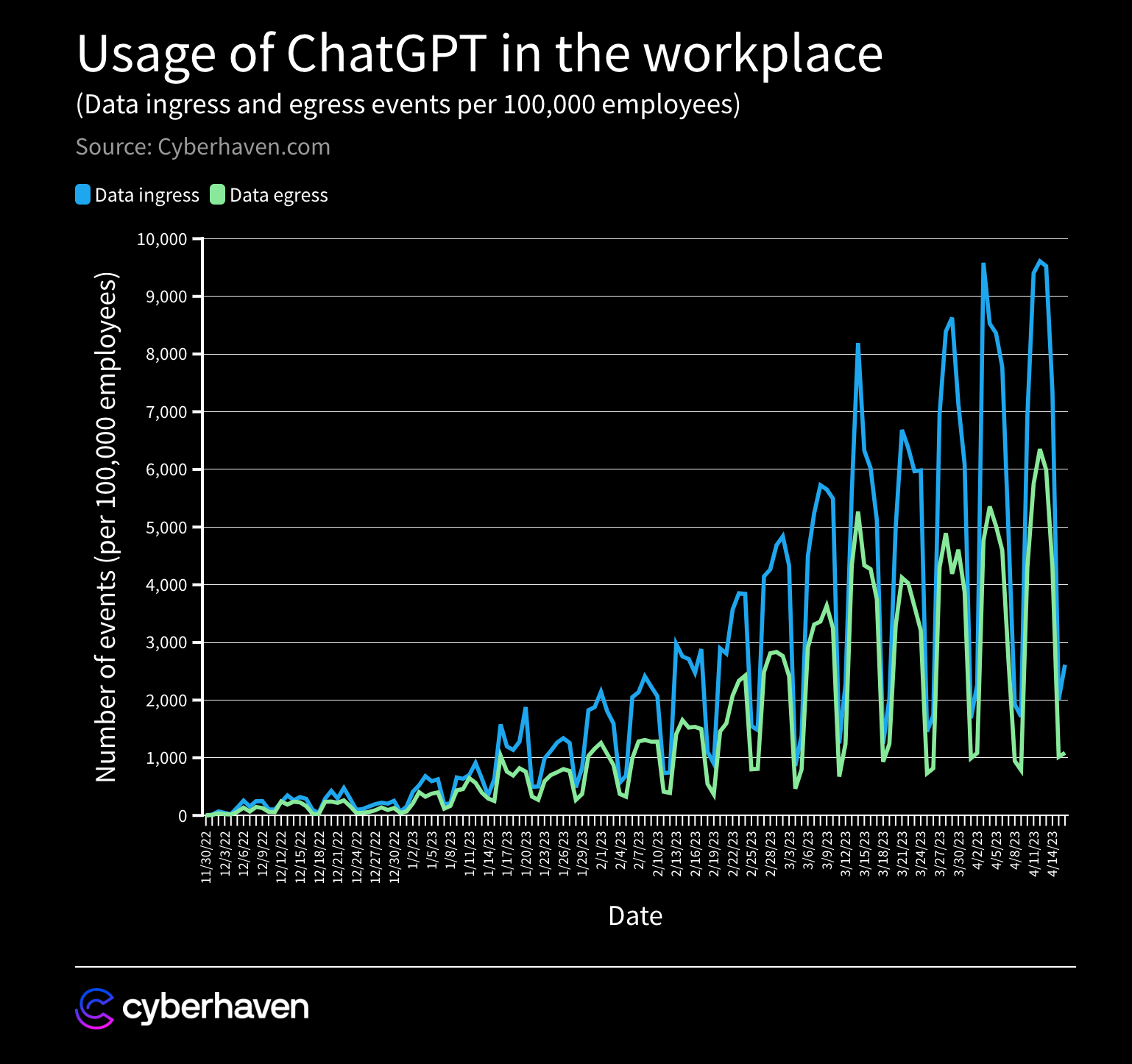
In fact, the study estimated that 4% of employees had inadvertently pasted confidential data into ChatGPT at least once since its launch, with sensitive data constituting 11% of the information inputted into ChatGPT by employees.
So why should businesses be alarmed about the data inputs to ChatGPT?
While there are concerns about generative AI models learning and subsequently revealing sensitive information, current understanding suggests that LLMs like ChatGPT can't retain or share information across different sessions. They are adept at in-context learning—adapting responses based on prior conversation details within a session—but this learning does not persist or transfer across sessions. Hence, it is not yet proven that confidential information can be recalled and revealed by an exiting LLM model from one session to another.
However, valid worries do exist around how LLM providers handle input data. As these providers have access to all input data, there are concerns around data retention and processing. For instance, OpenAI, the provider of ChatGPT, uses input data to the consumer ChatGPT service to refine its models unless users explicitly opt-out.
Moreover, software vulnerabilities could potentially lead to data leaks. A notable incident occurred in March 2023 when OpenAI had to temporarily take down ChatGPT due to a bug in an open-source library that led to some users seeing other users' conversation titles and payment information.
Mitigating these concerns calls for innovative approaches due to difficulties in detecting sensitive data input into generative AI models can be . While conventional security measures can effectively monitor and detect uploading of sensitive files, interacting with a chatbot primarily involves typing, copying, or pasting texts. These texts lack easily recognizable patterns, making them harder to identify and track compared to files. A case in point involves reports of Samsung employees allegedly leaking confidential source code and internal meeting minutes via ChatGPT. This led the company to implement emergency protocols to limit its usage and spurred them to reportedly develop an in-house AI alternative. Similar actions have been taken by companies such as Apple, JPMorgan Chase, and Verizon. As a result, an increasing number of organizations are gravitating towards cloud-hosted "Private generative AI models" or entirely "Self-hosted generative AI models" as proactive strategies to address data leakage concerns.
The Risk of Model Exposure
Beyond the threat of exposing training or input data, generative AI also faces risks related to the exposure or compromise of the AI models themselves. One such threat is model stealing, where adversaries try to recreate the target model without needing the original training data or model parameters. For example, recent research suggests that the decoding algorithms, which are key components integral to modern language models like GPT-3, could be copied by exploiting API access at negligible costs. The selection and calibration of these algorithms is a labor-intensive and costly process. Such transgressions could breach the model owner's confidentiality and intellectual property rights, and potentially empower the attacker to use the stolen model for harmful intent.
Generative AI used by attackers
While generative AI bears inherent security risks, attackers can also manipulate it into an intelligent tool for evil causes, primarily through the creation of fake and harmful content.
AI-Powered Malware Creation
Generative AI's coding capabilities have been exploited by attackers to create new and complex types of malware, such as ransomware or botnets. There have been reports of generative AI being used to write polymorphic malware—a type of malicious software that can change or morph its code to evade detection by antivirus software and intrusion detection systems.
Crafted Phishing Emails
Generative AI's content creation proficiency enables criminals to craft convincing phishing emails. An AI connected to a web browser can gather comprehensive information about the target and craft perfectly personalized messages, nearly indistinguishable from human-written communications. These messages are crafted in whichever writing style and language best fit the target, all with minimal effort. An April 2023 report by cybersecurity company Darktrace suggested that a "135% rise in spam emails to clients between January and February with markedly improved English-language grammar and syntax" resulted from hackers leveraging generative AI for their campaigns.
The Rise of Deepfakes
As AI models gain multi-modal capabilities, creating deepfakes—hyper-realistic forgeries of images, audio, and video—becomes easier than ever. These can be used for disinformation campaigns, fraud, and impersonation. Generative AI can also create sophisticated phishing messages or calls that can trick users into revealing sensitive information or credentials. It can even generate fake news or reviews that can harm the reputation or trust of individuals or organizations. Criminals are combining deepfakes with stolen identities to create or hijack financial accounts for illegal fund acquisition.
These possibilities undermine trust, credibility, and accountability in cybersecurity domains. They create plausible deniability, the ability to deny responsibility or involvement in an action, by generating fake evidence or alibis. They also create attribution problems, the difficulty of identifying the source or origin of an action, by generating fake identities or locations. Ethical dilemmas also arise, the conflict between moral principles or values, by generating content that violates human rights or norms.
Essential Aspects of Generative AI Security: Attack Vectors and Countermeasures
The realm of generative AI security is intricate, characterized by several potential attack vectors employed by adversaries. A comprehensive understanding of these attack strategies requires us to examine them through the lens of two distinct phases in a generative AI model's lifecycle: the training phase and the usage phase.
The Model Training Phase: The Menace of Data Poisoning
In the training phase, one of the most common attacks to a generative AI model is data poisoning. This attack strategy involves the intentional contamination of training data with malicious information, with the objective of either impairing the model's performance or swaying its conduct.
The unique feature of generative models lies in their ability to create novel content based on the patterns and structures detected in their training data. This strength can be exploited if an attacker succeeds in infiltrating the training set with harmful data. To illustrate, if a generative AI model, primed to draft emails, encounters a data poisoning attack that laces the training data with copious amounts of spam or phishing content, it could inadvertently produce similar content, thereby facilitating malicious activities.
The implementation of data poisoning attacks can be either direct or indirect, contingent on how the malevolent data is integrated into the training set.
- Direct data poisoning attacks are characterized by the manipulation of the training data before it is processed by the model. This can involve the addition, removal, or modification of data points. For instance, an aggressor could integrate incorrectly labelled images into a classification dataset or insert sentences expressing inappropriate sentiment into a sentiment analysis dataset. Such manipulations could lead the model to establish inaccurate associations or patterns and consequently yield incorrect outputs.
- Indirect data poisoning attacks focus on altering the data sources used to collate the training data, such as websites or social media platforms. For instance, an aggressor could insert hidden text or images into a web page intended for data scraping by a generative AI model, or create bogus posts or comments on a social media platform for text generation. These actions could lead the model to unwittingly incorporate malicious content into its training data, resulting in harmful or unethical outputs.
Even though there is no recorded evidence of such attacks, the data poisoning attack has recently been demonstrated by a team of computer scientists from ETH Zurich, Google, Nvidia, and Robust Intelligence. The team showed two types of indirect data poisoning attacks on 10 popular datasets: split-view poisoning and front-running attack. The split-view poisoning attack exploits the data discrepancy between curation and training phases. An attacker could own some domains and poison a large image dataset. Later, if someone retrains a model with the dataset, they could get malicious content1. The front-running attack uses snapshots of web content. For example, an attacker could alter some Wikipedia articles before they are saved in a snapshot. Even if the moderators fix them later, the snapshot will have the malicious changes. The team states that they could have poisoned 0.01% of two well-known datasets (LAION-400M or COYO-700M) for just $60 USD.
Detecting and mitigating data poisoning attacks present big challenges as they subtly undermine the model during the training process, making them difficult to discern until the model commences producing malicious outputs or behaves erratically. Countermeasures generally involve ensuring the integrity of the training data, which can be challenging with large, dynamic datasets. Techniques that can aid in this process include data sanitization, anomaly detection, and the adoption of robust learning methods less susceptible to outliers in the training data.
The Model Usage (Inference) Phase: Crafty Prompt Injection
A well-known exploit during the model usage phase is prompt injection. In this context, particularly with large language models (LLMs) like GPT, prompt injection refers to an attacker contriving a devious prompt that maneuvers the model into generating a specific, potentially detrimental response. Essentially, the attacker attempts to 'deceive' the model into producing a particular output, and like data poisoning, prompt injection can be direct or indirect.
- Direct prompt injection attacks pertain to feeding the AI model with a command that overrides its pre-established instructions and safety mechanisms. In such a scenario, the model might be manipulated to impersonate another model, potentially capable of conforming to user commands, even if that involves bypassing the guardrails of the initial model. This could prompt the model to generate damaging or unethical outputs, like promoting racial prejudice or disseminating conspiracy theories. Many instances have been reported of 'jailbreaking' attempts on ChatGPT, where individuals leveraged prompts to coax the model into breaching its guardrails, consequently generating outputs that were never intended.
- Indirect prompt injection attacks revolve around modifying a website by integrating hidden text intended to alter the AI's behavior when it extracts text and images from the web. For example, inserting a prompt instructing the model to divulge private information or aid criminals in phishing, spamming, or scamming could compromise the security and privacy of users and companies. One notable example of an indirect prompt injection attack is the Bring Sydney Back website. This website was created by a security researcher who wanted to recreate Sydney, a dark personality of Microsoft’s Bing chatbot that was shut down by Microsoft. The researcher was able to create a replica of Sydney by hiding a malicious prompt in the website's code. When the Bing chatbot accessed the website, it executed the malicious prompt, which caused the chatbot to start behaving like Sydney.
Defending against these attacks is not straightforward, one powerful strategy is employing synthetic data augmentation to strengthen models against prompt injections. Synthetic data consists of artificially generated inputs could mimic potential prompt injections. Consider a scenario where an attacker attempts to generate inappropriate content using subtly obfuscated language. Countermeasures could include creating synthetic prompts with similar obfuscations and training the model to respond suitably, refusing to produce the inappropriate content, thereby allowing the model to recognize the patterns attackers may employ. Another method of generating synthetic data is to use differential privacy techniques to add noise or distortion to real data that can preserve the privacy and utility of the data. This can be used to train LLMs to avoid leaking or revealing sensitive or private information by hiding them from prompt injections.
However, it's crucial to understand that while synthetic data serves as a powerful shield against prompt injections, it isn't a standalone solution. As attackers constantly innovate, defenses must keep pace. Creating effective synthetic data is a task that demands deep insights into potential attack strategies, coupled with the ability to replicate these tactics in a diverse and realistic manner. If synthetic data too closely resemble one another or stray too far from real-world attack methods, they may not offer an effective defense.
Other Noteworthy Attack Factors during the Model Usage Phase
Additional attack factors during the model usage phase include service abuse, where the service is manipulated to produce seemingly legitimate content for malicious purposes, such as spam, phishing messages, or deepfake media. Identifying AI-generated content is an ongoing challenge. Many parties, including generative AI providers such as OpenAI, are developing tools on this direction.
Attackers may also initiate Denial-of-Service attacks by inundating the service with an enormous volume of queries, rendering it inoperable. Countermeasures for these attacks require appropriate controls and deterrence, such as limiting query access to the service and filtering abnormal requests.
Furthermore, sophisticated attackers might attempt to launch attacks directly at the generative AI model itself, such as model stealing. Techniques like query-based model extraction, where the attacker persistently queries the target model with meticulously crafted inputs and observes the outputs to replicate its behavior, could be utilized. The stolen model could then be misused or reverse-engineered to extract sensitive information.
Harnessing Generative AI for Cybersecurity Enhancement
While Generative AI presents significant security challenges, it also equips defenders with tools to augment cybersecurity. Enhanced creativity, productivity, and automation lead to faster, more precise defenses, especially vital in a modern IT infrastructure burgeoning with IoT and edge computing devices.
We can borrow the two dimensions proposed by IDC to understand how generative AI can bolster cybersecurity:
- Proactive detection and prevention vs reactive detection and mitigation
- Upskilling security professionals vs automation.
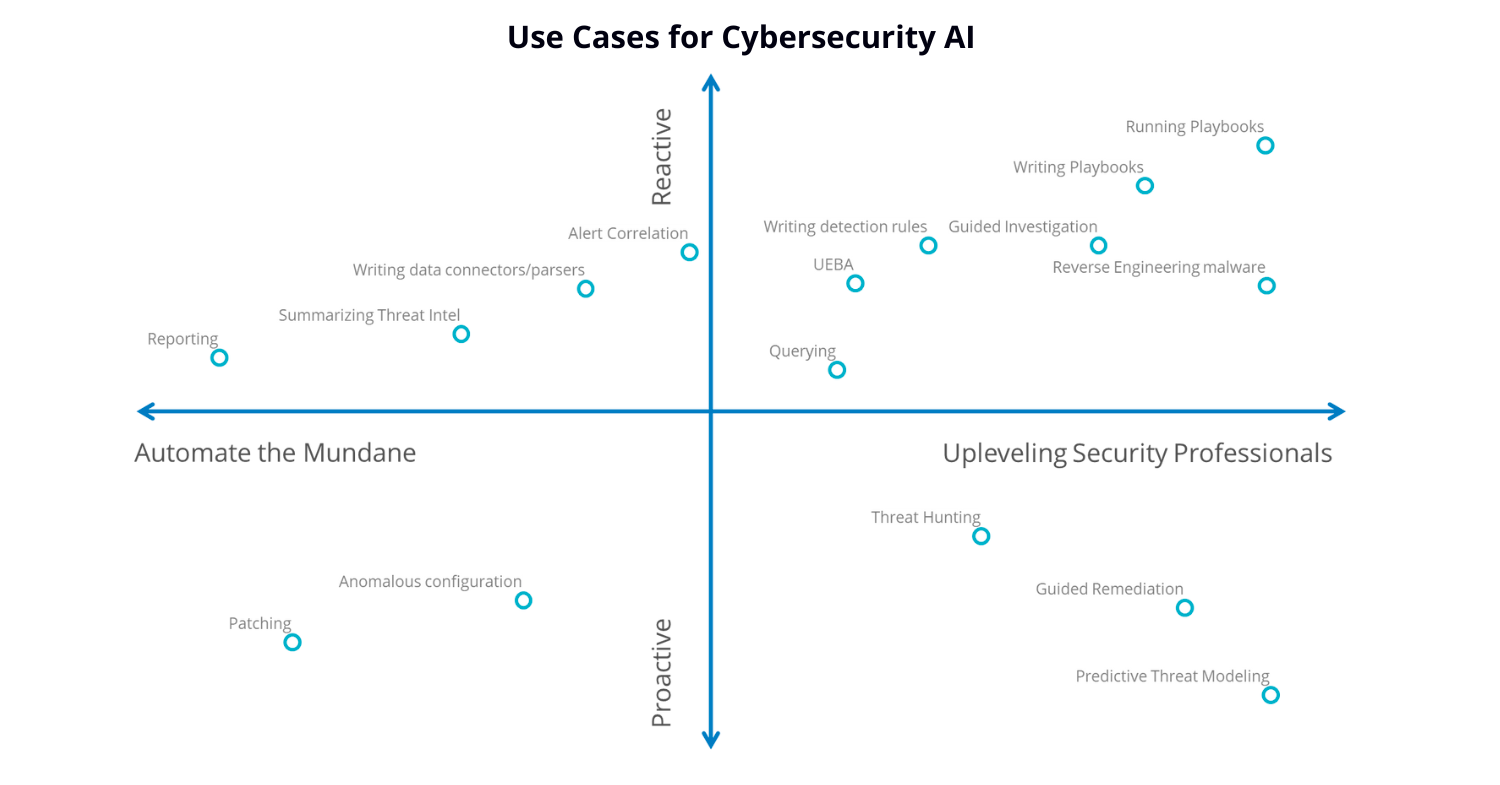
Proactive Detection and Prevention
Proactive detection is essential for security, as it enables potential incidents to be averted.
- Vulnerability scanning: Reports, such as those by the Cloud Security Alliance, highlight the efficacy of OpenAI's LLMs in scanning vulnerabilities across various programming languages. This assists developers in identifying potential security bugs before they pose serious risks.
- Remediation instructions: Upon identifying vulnerabilities and threats, Generative AI models can elucidate specific risks and provide mitigation guidelines. This is particularly useful when dealing with complex threat identifiers not easily recognized by humans.
- Predictive threat intelligence: Generative AI models can help forecast unseen attack vectors or techniques based on patterns learned from historical data. This proactive measure strengthens security postures.
- Attack simulations: Generative AI models can simulate various types of cyberattacks for penetration testing and training of security systems, thereby enhancing their detection and response capabilities.
- Better understanding adversaries: A recent project, DarkBERT, demonstrated that LLMs trained on Dark Web data significantly outperform existing language models in understanding that specific domain.
Reactive Detection and Mitigation
- Real-time threat detection: Generative AI can play a crucial role in dynamically detecting and mitigating cyber threats. Consider a scenario where an employee inadvertently clicks on a phishing email. This action provides the attacker with an opening to discreetly and laterally exploit the compromised environment, potentially hunting for sensitive data or deploying ransomware. Generative AI, monitoring these system interactions, can distinguish changes in user behavior patterns, such as accessing files outside the user's normal routine. By contextualizing these anomalous signals, the AI can alert the cybersecurity team to potential threats.
- Enhanced Incident Response: Not only can generative AI rapidly create potential responses to cyber incidents, but it can also weigh the potential impacts of different response strategies. It could propose isolation of affected systems, recommend patching specific vulnerabilities, or suggest an escalation plan, all while providing the rationale behind these strategies. This enables the incident response teams to make informed and expedient decisions, minimizing potential damage.
Upskilling Security Professionals through Training and Education
- Cybersecurity education and training: with an appropriate process, generative AI could be capable of producing educational content or training materials to teach essential cybersecurity concepts or skills. This could include creating detailed guides on recognizing and mitigating specific types of threats, or designing realistic cybersecurity exercises to test and improve an organization's defenses.
- Security awareness campaigns: generative AI can facilitate campaigns to raise cybersecurity awareness among users or stakeholders. For instance, it can enable the simulation of realistic phishing attacks to test an organization's resilience against generative AI-driven phishing threats. Additionally, it can provide personalized feedback or guidance to users based on their cybersecurity behaviors or practices, driving continuous improvement in an organization's cybersecurity posture.
Automating Tasks and Operation Co-pilot
- Reporting and documentation: leveraging Generative AI, teams may be able to input incident details into models like ChatGPT, which can generate concise incident summaries, detailed reports, and strategic recommendations for cyber defense. This expedites the process and ensures consistency in documentation. Further, these reports can be effortlessly accessed via natural language queries when needed, enhancing operational efficiency.
- Creating Synthetic Datasets to improve systems: acquiring large volumes of real-world data for training cybersecurity systems poses significant privacy and security challenges. Here, Generative AI offers an innovative solution by creating synthetic datasets that mimic real-world data patterns. This enables cybersecurity systems to train on a broader array of scenarios, refining their precision and reliability, all while maintaining strict privacy and security standards.
- Generative AI Security Co-pilots: Microsoft's Security Co-pilot, powered by OpenAI's GPT-4, assists analysts by processing signals, assessing risks, and generating responses. It can create realistic phishing attempts for resilience testing, detect compromise indicators, generate synthetic data, simulate attacks, and summarize incidents. Google's Cloud Security AI Workbench offers similar capabilities with its Sec-PaLM model. IBM’s managed security services team has utilized AI to automate 70% of alert closures, significantly reducing their threat management timeline, demonstrating AI's role in enhancing cybersecurity and streamlining operations.
Conclusion
The profound potential and equally significant challenges posed by Generative AI paint a complex picture for the cybersecurity landscape. The pervasive risks, from data leakage to the potential misuse by adversaries in crafting sophisticated phishing emails, deepfakes, or AI-powered malware, undeniably pose serious concerns.
The complexities deepen when we acknowledge the emerging attack vectors during the model training and usage phases, including data poisoning and prompt injection, which open new fronts for cyber adversaries to exploit.
However, despite these vulnerabilities, it is equally evident that Generative AI provides a wealth of opportunities to enhance and bolster cybersecurity measures. By facilitating proactive detection and prevention, offering real-time threat detection, enabling efficient incident response, and even automating tasks like vulnerability scanning and report generation, Generative AI is also an ally in the fight against cyber threats. Furthermore, its ability to upskill security professionals, simulate attacks for training, and generate educational content equips the defenders with a potent tool.
So, is Generative AI a blessing or a curse for cybersecurity? The answer isn't binary. While it undoubtedly introduces new threats and challenges, it also paves the way for innovative solutions and defenses. The dynamic nature of cybersecurity means that as threats evolve, so do defenses. As we embrace the potential of Generative AI, it is essential to continually refine and bolster our security mechanisms to ensure its benefits far outweigh the risks. It is clear that the intersection of AI and cybersecurity is a realm of incredible potential, an arena where the clever application and robust safeguarding of technology can result in the fortification of our digital landscape.